We have published a paper in the Journal of Petrology describing a novel approach to quantifying the distribution of atoll garnet in a metamorphic rock using micro-computed tomography and deep learning algorithms capable of automated textural analysis of crystal shapes in 3D. We show that this method can be applied to study the kinetics of fluid-driven metamorphic reactions such as atoll garnet formation, and propose a kinetic model to calculate the reactivity of metastable garnet to form atolls at different pressure and temperature conditions.
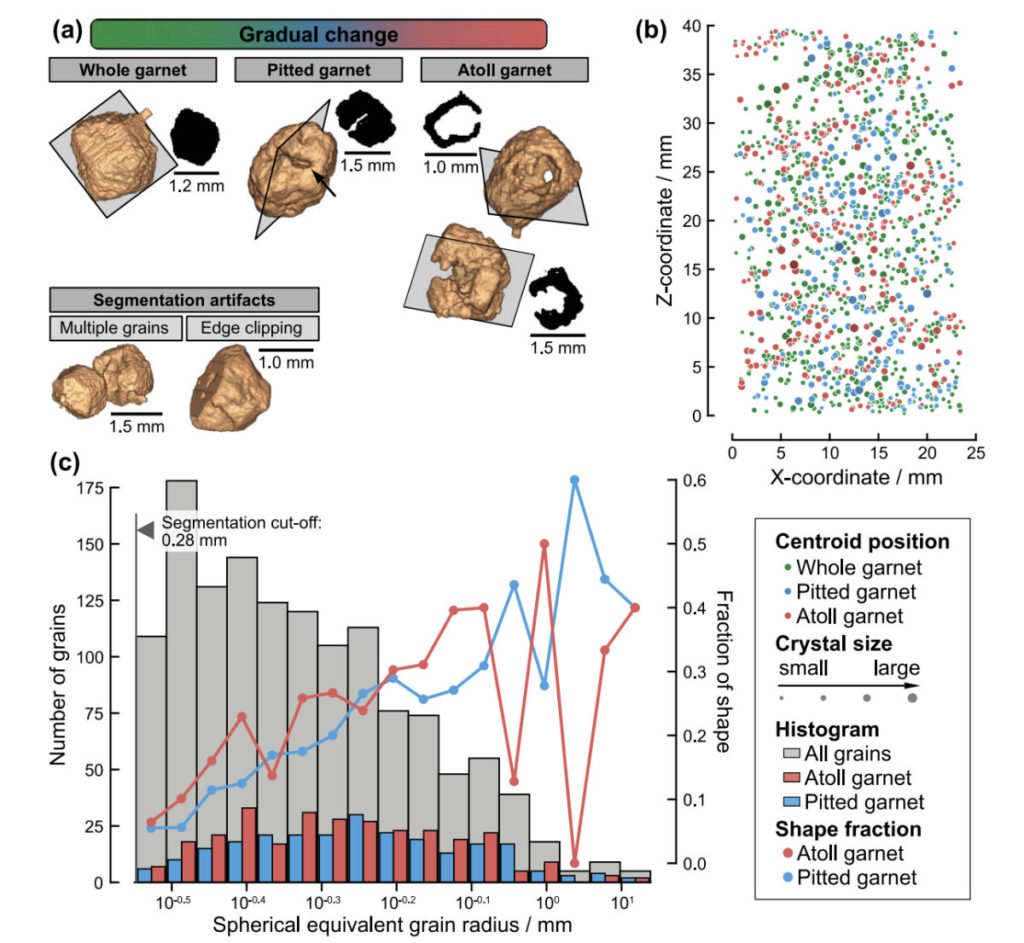
convex hull that fits the grain. The x-axis shows the grain size as spherical equivalent radius on a logarithmic scale. A second axis shows the fraction of grains in each size category that are atoll (red) and pitted (blue). Segmentation cut-off of 0.28 mm is highlighted by the vertical line.
Find out more
Hartmeier, P., Lanari, P., Forshaw, J., Markmann, T.A. (2024). Tracking garnet dissolution kinetics in 3D using deep learning grain shape classification. Journal of Petrology, 65, 1-9. Download pdf | Visit the journal webpage